Animals are able to attach simple ratings to complex objects in their environment to guide behavior. For example, humans can easily tell whether they like a wine or not, which will influence their future choices in the wine store. Similarly, animals can tell whether a complex odor is good or bad in order to decide whether to approach or avoid it. This ability to assign behaviorally relevant value to complex objects is essential for intelligent behavior. Researchers from the Friedrich group revealed how odors are associated with “good” or “bad” in the brain of zebrafish, and how these associations are modified during learning. Surprisingly, they find that inhibitory neurons make a major contribution to the specificity of these associations.
The group of Rainer Friedrich studies neuronal computations and their experience-dependence, using zebrafish as a model system; the small vertebrate provides important advantages for mechanistic analysis of circuit structure and function. One focus of research for the group is Dp, a brain area that is homologous to the mammalian olfactory cortex and thought to store memories in an auto-associative network.
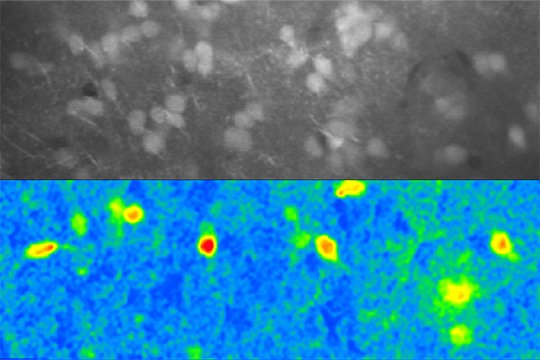
[ad_336]
Thomas Frank, a postdoc in the Friedrich group, had two goals in mind when he started his project: to understand how Dp stores memories, and to explore how inhibitory neurons contribute to this process. He trained adult zebrafish in an odor discrimination task and measured activity patterns in a dorsal-posterior subregion of Dp (dpDp) that had not been characterized in detail before. With their study, which has just been published in Nature Neuroscience, Frank and colleagues made two major findings.
First, the researchers found that responses of neurons in dpDp to odors were enhanced after associative conditioning. Largest enhancements were observed for those odors that were associated with a food reward. These results are generally in line with simple models of associative learning. However, patterns of activity were not only stronger after learning but also changed in more complex ways. Further analysis showed that activity patterns in dpDp contain information about the identity of an odor, and also about its valence – which means whether it is “good” or “bad”. In fact, much of the neuronal activity in dpDp represented valence in a rather simple fashion. The researchers then found that activity patterns in dpDp were “twisted” systematically when the valence of odors was manipulated in an experiment. For example, when researchers made an odor more attractive by associating it with a reward, odor-evoked activity patterns changed so that their valence code looked more “positive”. Subtle differences in this valence code even predicted small differences in the behavior of individual fish, indicating that the valence code is indeed behaviorally relevant. dpDp is therefore a brain region where complex odor information is directly associated with “good” or “bad”.
[rand_post]
The second main finding concerns the role of inhibitory neurons. Models of memory networks often assume that learning is mediated by specific modifications of excitatory synaptic connections, whereas inhibitory connections do not play a major role. However, the function of inhibitory neurons had not yet been analyzed in detail. To address this issue, Frank expressed a molecular tool in inhibitory interneurons to reversibly shut down their activity with yellow light. This allowed him to compare odor-evoked activity in the same fish when inhibitory neuronal networks were functional or silent. Surprisingly, he found that some of the plasticity that occurred during learning was reversed by silencing inhibitory interneurons. These results show that specific changes in inhibition also make important contributions to associative learning.
“These results reveal a neuronal circuit that gives value to sensory stimuli,” says Rainer Friedrich. “This is an important step to make good decisions. In addition, we now know that inhibition has much more sophisticated functions in memory networks than expected.”
Friedrich also highlights that the project became possible only because multiple team members contributed different expertise, and because Frank developed a series of new tools.
The group leader concludes: “This was a highly demanding project, and Thomas did an outstanding job. He has just started as a group leader at the MPI in Martinsried and I wish him all the best and lots of fun!”